Supervised by: Dr Callum Parr MBBS BSc (Hons). Callum studied Medicine at Imperial College London and secured a place as an Academic Foundation Doctor in the competitive North West London region. He received multiple distinctions for his performance throughout medical school. He intercalated in Biomedical Engineering, also at Imperial College London, and received First Class Honours. He has published work in prestigious journals, including the Lancet Respiratory and BMJ.
Abstract
The field of radiology involves the diagnostic testing and imaging of various parts of the body. Traditionally, the images and diagnoses would be primarily analysed by trained physicians; a visual assessment of the medical images would be conducted, and physicians would note any characterisations of pathologies (1). However, diagnoses made by humans can often be varying, incorrect, and unreliable; this is why the use of artificial intelligence (AI) is rapidly growing in medicine and healthcare. In contrast with physicians, AI demonstrates promising improvements in the efficiency and accuracy of radiology. AI is capable of processing large amounts of patient data and images, and recognising complex algorithms and radiographic characteristics among the data (1). As it stores past patient histories, AI also demonstrates its ability to supplement and improve diagnostic intelligence, serving as a “second opinion” to physicians (2). As AI increases its presence in the various fields of medicine, researchers continue to learn about the benefits and drawbacks it brings.
Introduction
X-ray scans are the most popular technique of imaging due to multiple factors, including their low cost and speed. These images are produced non-invasively by passing a series of electromagnetic waves (at various wavelengths, depending on the scan test) through the body’s tissues and detecting the attenuated rays on the other side (3). For an x-ray, images are produced through high-energy radiation at shorter wavelengths, and the images are projected in 2D form with a resolution down to 100 microns (4). They can be utilised to detect and diagnose numerous conditions such as breast cancer, bone fractures, osteoporosis, and more (3).
In general, artificial intelligence excels in performing and automating repetitive tasks, such as image analysis and pattern recognition. In radiology, computer systems are fed a large variety of data until an effective algorithm is formed; the data input can either be in numerical form (i.e. heart rate, blood pressure, stroke volume, pH levels, etc) or image-based (i.e. an x-ray) (5). A large amount of data is required to expose the algorithm to various conditions and to ensure accuracy in the analysing process, until eventually, AI will be able to differentiate healthy scans from abnormal scans.
Aid in Diagnosis
The earliest applications of AI for chest radiograph analysis involved the detection of lung nodules – small, abnormal swellings found inside the lungs6. However, an increasing number of cases show how AI can also be used for more complex diagnoses, such as pneumothorax detection, pleural effusion detection, tuberculosis screening, and other chest pathologies (6).
Example 1: Pneumothorax Detection
A pneumothorax (PTX) is a collapsed lung that occurs when air from the lungs leaks into the pleural cavity – located between the lungs and the chest wall. When this occurs, the pressure within the pleural cavity will become greater than the pressure within the lung, causing the lung to collapse (7). Any case of pneumothorax is potentially life-threatening; therefore, it is critical to treat the condition immediately upon detection.
Either supine or erect chest radiographs (CXR) may be used to image pneumothorax. Erect CXRs are the standard position, where the patient is upright with their back against the image receptor; meanwhile, supine CXRs are taken while the patient is lying back against the image receptor, and the x-ray machine scans from front to back. It is important to note that the supine position is inferior due to its limitations in capturing accurate heart size, mediastinal contours and width, lung inflation, and diaphragm contours (8). These radiographs are then annotated for PTX size and location (i.e. unilateral or bilateral), as well as inserted thoracic tubes (9). Thoracic tube insertions are found to be confounding variables that can potentially limit and bias established pneumothorax-detecting artificial intelligence algorithms (9). The final in-image pixel annotations of the PTX-positive data are fed into computer software and translated into AI algorithms (9). In this manner, AI learns to recognise the varying sizes and locations of PTX-positive chest scans.
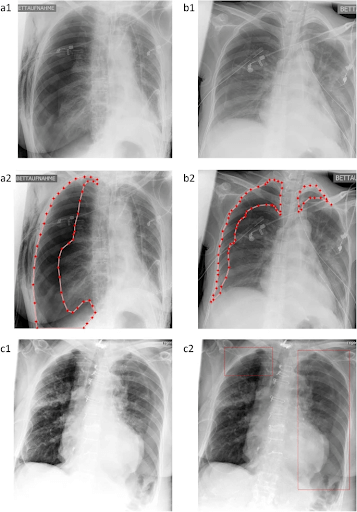
Fig. 1 – Chest x-rays show annotations of dehiscences in the visceral pleura. (a2, b2): The pixel coordinates of the damaged visceral pleura and thoracic wall are annotated by physicians, and they are then used for algorithm training. (c1, c2): “Algorithm 2” demonstrates the algorithm confidence of AI in locating PTX in the SCXR. (c1) Original contrast-enhanced DICOM, (c2) AI-finding enriched image (9).
Example 2: Tuberculosis Detection
Substantial progress has also been made towards developing AI algorithms for tuberculosis screening, with certain potential applications achieving near 100% in sensitivity and accuracy. For example, an ensemble of deep conventional networks by AlexNet and GoogLeNet have demonstrated an area under the receiver operating characteristic (AUROC) of 0.99 in their ability to properly screen, diagnose, and classify chest x-rays as exhibiting pulmonary manifestations of tuberculosis or not (6). In another analysis conducted in Dhaka, Bangladesh between May 15, 2014 and October 4 2016 (10), chest x-rays from 23,954 individuals were screened for symptoms of tuberculosis – first through a verbal screening and a standard digital posterior-anterior chest x-ray, followed by an Xpert MTB/RIF test. All x-ray images were read independently by three registered radiologists, as well as five commercial AI algorithms: CAD4TB (version 7), InferRead DR (version 2), Lunit INSIGHT CXR (version 4.9.0), JF CXR-1 (version 2), and qXR (version 3) (10). Again, all five AI algorithms significantly outperformed the team of radiologists; while maintaining a sensitivity level above 90%, the five algorithms also reduced the number of Xpert tests required by 50% (10).
Tuberculosis (TB) is an infectious disease caused by the Mycobacterium tuberculosis bacteria. This bacteria is airborne and primarily affects the lungs, and it is spread from person to person through coughs, sneezes, and other expelled droplets containing the TB bacteria. In general, to find inactive TB, AI algorithms will be scanning for TB-specific abnormalities in x-rays, including pleural thickening, persistent calcification, a persistent tuberculoma, and general lung volume loss (11). In active post-primary cases of pulmonary tuberculosis, either it is TB reactivation or secondary TB, x-ray findings will also show an ill-defined, hazy consolidation with a cavitary lesion or fibroproliferative disease; these findings usually involve the posterior segments of the upper lobe, or the superior segment of the lower lobes spread to within the bronchi (11).
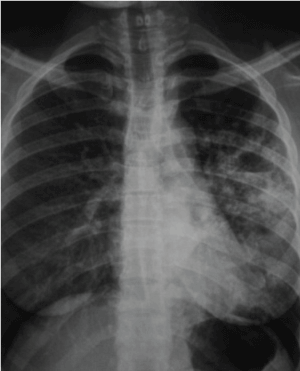
Fig. 2 – Chest x-ray shows cavitary lesion and air-fluid level on the lower left and middle lobe of right lung, post-primary pulmonary TB (11).
Certain AI applications, such as the CAD4TB, will scan for these TB-specific abnormalities in the chest x-ray and output continuous abnormality scores ranging from 0 to 100 (12). If an abnormality is found, the score is increased; the greater the final abnormality score, the higher the probability of tuberculosis. However, diagnosing tuberculosis solely through scanning for TB-specific abnormalities is not reliable, since many other lung pathologies present with similar radiological patterns (12); therefore, chest x-rays are yet only of limited use for diagnosing tuberculosis. Instead, the scan tests will usually be performed in conjunction with other laboratory tests to ensure accuracy in the diagnosis.
Benefits of the Use of AI in X-Rays
AI wields the ability to be ground-breaking in many fields, including radiology. The use of AI can help in improving a plethora of different factors of radiology, the primary of which is efficiency (3). Compared to medical staff and radiologists, AI is able to interpret x-rays at a significantly faster speed. In a study done by Stanford University researchers, it was found that AI is capable of simultaneously scanning 14 different pathologies (13). This is much faster than a human radiologist, who would have to go through each x-ray one at a time, taking long periods of time to analyse and identify different pathologies. AI does not slow down over time, as humans tend to do, which means that it will be able to continue scanning x-rays at a constant, high speed for as long as necessary. The ability to handle multiple pieces of data at once allows it to classify and separate data quicker than medical specialists (3). Furthermore, the accuracy of radiograph analysis has the potential to be enhanced by AI (3). The use of AI eliminates the factor of human error that can cause scanning and interpretation of radiographs to be incorrect, thus possibly leading to incorrect diagnosis. This is because AI compares the x-rays to the datasets that they have been programmed with, in order to find similarities and differences between them, which means that they will be able to identify specific parts of x-rays which indicate diseases based on previous proof. This is a benefit as it means that errors are highly unlikely due to the fact that every interpretation is backed up by evidence from a case that occurred prior to the analysis. Finally, AI can also greatly elevate the reliability of radiographic analysis as compared to radiologists (3). This is because, once again, human error is avoided, and so AI will be able to attain similar results every time it scans an x-ray. On the other hand, if two radiologists were to analyse the same radiograph, they may interpret the scans to show different diseases, or one may not identify any disease.
Complications Regarding the Use of AI in X-Rays
Although AI is already making several successful advances in radiology and has incredible future prospects, there are several concerns regarding the use of this technology, especially in the field of radiology. First of all, it is not always easy to understand what methods AI tools are performing, and the reasons behind these decisions. This makes it difficult for us to control and adjust them, especially as these apparatus are incessantly learning and ever-changing (4). Secondly, AI algorithms must be constantly adapted, and AI tools regularly tested and modified, in order for their analyses to be relevant (4). Furthermore, AI currently does not succeed well in interpreting cases that are one-off as it does not have prior data sets to compare it to. A similar issue will arise for variant diseases and pathologies that AI is not programmed to scan for; it will not detect them or inform the relevant specialist of the disease, which could be potentially life-threatening for patients. As of now, AI is still in the process of development, and whilst it is already being utilised, its full potential has not been reached. This means that its current ability is limited and not of as much benefit to radiology as it can and will be in the future.
The Ethics of AI in Radiology
As the contribution of AI continues to grow in the field of medicine, debates about the ethical issues behind it are also on the rise. The complexity of AI is raising questions and concerns on how AI algorithms are developed and used. In particular, radiology is a heavily data-driven specialty (14); therefore, ethical issues concerning data ownership and privacy are of primary concern. In order to establish, train, and perfect AI-based algorithms, AI must have access to a large volume of patient data (15). Here, ethical issues surrounding data ownership, privacy of data, and how the data will be used arise; however, increasing legal restrictions are being established in healthcare systems around the globe. The European Union General Data Protection Regulation (GDPR) has established that patients have complete control over their own identifiable and sensitive data (15). Furthermore, patients must also give explicit consent for the sharing and re-use of their personal data. Under the GDPR, patients must also give consent if their imaging data is to be used in AI algorithm training.
The increasing legal restrictions have potential to inhibit AI research in certain parts of the world, and may skew the global academic, research, and commercial competition (15). However, the restrictions are necessary, from a human rights standpoint. Ultimately, the key to success of AI integrations in the field of medicine is that the patient understands that AI is a tool being used for their safety and benefit.
Overall, AI has demonstrated great potential in improving multiple sectors in the field of radiology. It is able to diagnose and suggest treatments for diseases at speeds that human specialists cannot achieve, and progress in its development is rapid. Although AI comes with many complications at present, there is no doubt that with its ability to continuously adapt and learn, these issues will be overcome.
Bibliography
- Hosny A, Parmar C, Quackenbush J, Schwartz L, Aerts H. Artificial intelligence in radiology. Nature Reviews Cancer. 2018 Aug;18(8):500-510. DOI: 10.1038/s41568-018-0016-5.
- Summerton N, Cansdale M. Artificial intelligence and diagnosis in general practice. British Journal of General Practice. 2019; 69 (684): 324-325. DOI: 10.3399/bjgp19X704165
- Panayides AS, Amini A, Filipovic ND, Sharma A, Tsaftaris SA, Young A, Foran D, Do N, Golemati S, Kurc T, Huang K, Nikita KS, Veasey BP, Zervakis M, Saltz JH, Pattichis CS. AI in Medical Imaging Informatics: Current Challenges and Future Directions. IEEE Journal of Biomedical and Health Informatics. 2020;24(7):1837–57. DOI: 10.1109/JBHI.2020.2991043.
- Driver CN, Bowles BS, Bartholmai BJ, Greenberg‐Worisek AJ. Artificial Intelligence in Radiology: A Call for Thoughtful Application. Clinical and Translational Science 2020;13(2):216–8. DOI: 10.1111/cts.12704
- Greenfield D. Artificial Intelligence in Medicine: Applications, implications, and limitations. Science in the News. 2019. https://sitn.hms.harvard.edu/flash/2019/artificial-intelligence-in-medicine-applications-implications-and-limitations/
- Adams SJ, Henderson RDE, Yi X, Babyn P. Artificial Intelligence Solutions for Analysis of X-ray Images. Canadian Association of Radiologists Journal. 2020;72(1): 60–72. DOI: 10.1177/0846537120941671.
- Zarogoulidis P, Kioumis I, Pitsiou G, Porpodis K, Lampaki S, Papaiwannou A, Katsikogiannis N, Zaric B, Branislav P, Secen N, Dryllis G, Machairiotis N, Rapti A, Zarogoulidis K. Pneumothorax: from definition to diagnosis and treatment. Journal of Thoracic Disease. 2014 Oct; 6(Suppl 4): S372–S376. DOI: 10.3978/j.issn.2072-1439.2014.09.24
- Murphy, A. Chest (supine view). Radiopaedia.org. 2016 May. DOI: 10.53347/rID-45309
- Rueckel J, Huemmer C, Fieselmann A, Ghesu F-C, Mansoor A, Schachtner B, Wesp P, Trappmann L, Munawwar B, Ricke J, Ingrisch M, Sabel B. Pneumothorax detection in chest radiographs: optimising artificial intelligence system for accuracy and confounding bias reduction using in-image annotations in algorithm training. European Radiology. 2021; 7888–7900. DOI: 10.1007/s00330-021-07833-w
- Qin ZZ, Ahmed S, Sarker MS, Paul K, Adel ASS, Naheyan T, et al. Tuberculosis detection from chest x-rays for triaging in a high tuberculosis-burden setting: an evaluation of five artificial intelligence algorithms. The Lancet Digital Health. 2021;3(9): e543–e554. DOI: 10.1016/S2589-7500(21)00116-3.
- Al Ubaidi BA. The Radiological Diagnosis of Pulmonary Tuberculosis (TB) in Primary Care. Clinmedjournals.org. 2022;4(1). DOI: 10.23937/2469-5793/1510073.
- Qin, Z.Z., Sander, M.S., Rai, B. et al. Using artificial intelligence to read chest radiographs for tuberculosis detection: A multi-site evaluation of the diagnostic accuracy of three deep learning systems. Sci Rep 9, 15000 (2019). DOI: 10.1038/s41598-019-51503-3
- Armitage H. Artificial intelligence rivals radiologists in screening X-rays for certain diseases. News Center. Stanford Medicine; 2018. https://med.stanford.edu/news/all-news/2018/11/ai-outperformed-radiologists-in-screening-x-rays-for-certain-diseases.html
- Zoga A, Syed A. Artificial Intelligence in Radiology: Current Technology and Future Directions. Seminars in Musculoskeletal Radiology. 2018;22(05):540–5. DOI: 10.1055/s-0038-1673383
- Brady AP, Neri E. Artificial Intelligence in Radiology—Ethical Considerations. Diagnostics. 2020;10(4): 231. DOI: 10.3390/diagnostics10040231