Supervised by: Chi Him Kendrick Yiu BSc (Hons), MRes. Kendrick is studying for a DPhil in Medical Sciences at Wolfson College, University of Oxford, specialising in cardiovascular research. He graduated with a Bachelor’s Degree in Biomedical Sciences in Hong Kong before pursuing a Master’s Degree in Clinical Research at Imperial College London. His interests lie in the mechanisms of heart diseases and their potential therapies.
ABSTRACT
Background: Atrioventricular septal defects (AVSD) are the most prevalent of all congenital heart diseases (CHDs), consisting of almost 7% of diagnosed CHD worldwide. It is an irreversible congenital disease that must be detected early to ensure preventive methods are in place.
Results: Prenatal scans like fetal echocardiography have had significant improvements in the accuracy, sensitivity and specificity of their outcomes due to the use of artificially intelligent (AI) techniques including machine learning, supervised learning and deep learning. This has therefore led to a decreased number of patients with AVSD that have not been diagnosed yet, and an increase in the accurate identification of CHD cases owing to the invention of fetal intelligent navigation echocardiography (FINE) and other relevant techniques that are explored in this paper.
Conclusion: AI is effective in improving the accuracy of prenatal AVSD detection. Machine learning has aided the evolution of fetal echocardiography as a manual scan is no longer required. Furthermore, deep learning has been introduced in prenatal scans such as abdominal and transvaginal scans, results from which are important in the diagnosis of AVSD.
This review highlights the impact of AI in diagnosing AVSD. The paper aims to create a broader understanding of AI applications in pediatric cardiology for improved clinical practice, evaluate different diagnostic and prognostic approaches of AVSD, explore different AI techniques and their use in cardiovascular scans, and identify the use of AI in prenatal scans in different trimesters. The outcomes may drive further research and development of AI into the realm of early AVSD detection.
Keywords: congenital heart defects, machine learning, cardiovascular imaging, prenatal detection, deep learning, supervised learning, expert systems.
1. INTRODUCTION
Atrioventricular septal defect (AVSD), also known as atrioventricular canal (AV canal) defect, is a rare congenital heart defect (CHD) involving abnormal holes between the left and right sides of the heart and irregular formation of heart valves. AVSD only affects approximately 0.024% to 0.031% of live births (Ahmed and Anjum, 2023). AVSD can be further categorized into two types, complete AVSD and incomplete AVSD, based on the severity. A complete AVSD is present when the septa dividing the atria and ventricles fail to grow to the center of the heart and the common valve does not develop into the tricuspid and mitral valves (Centers for Disease Control and Prevention, 2023). In comparison to complete AVSD, in incomplete AVSD, a hole is often identified near the center of the heart and a valve is usually not fully closed (Centers for Disease Control and Prevention, 2023). Both variations of AVSD involve irregular blood flow in the heart.
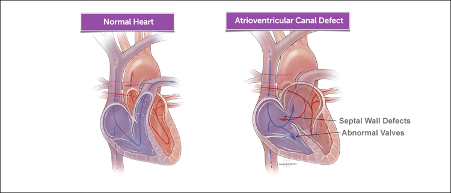
Figure 1. On the left shows the typical path of blood flow in a normal heart in comparison to an abnormal heart with AV canal, the alternative name of AVSD (Boston Children’s Hospital).
The signs of AVSD include heart murmur, breathing problems, pounding heart, weak pulse, ashen or bluish skin color, poor feeding, slow weight gain, tiring easily, and swelling of the legs or stomach. Worsening AVSD may lead to symptoms such as congestive heart failure, arrhythmia, and pulmonary hypertension (Centers for Disease Control and Prevention, 2023). The Centers for Disease Control and Prevention (2023) further explained that for partial AVSDs, if the holes between the chambers of the heart are not large, the signs and symptoms may not occur in the newborn or infancy periods. In these cases, people with a partial AVSD might not be diagnosed for years. Early detection of the condition is crucial, especially for asymptomatic patients, to avoid and prevent severe symptoms of AVSD.
Echocardiograms, specifically fetal echocardiograms, is a common medical imaging test used for the prenatal diagnosis of AVSD. However, the unstable accuracy of prenatal diagnosis is an ongoing problem as the failure of early detection of CHD decreases the survival of infants with the severe condition (Day et al., 2021). As stated by Reddy, Eynde, and Kutty (2022) from the National Library of Medicine, there is potential improvement to numerous areas of fetal echocardiography, namely “[improving] image acquisition, image optimization, automated measurements, identification of outliers, classification of diagnoses, and prediction of outcomes”, through the implementation of artificial intelligence (AI).
AI has become a powerful tool for medical imaging, owing to the development of its algorithms with the potential to learn, reason and self-correct in a human-like manner. (Reddy, Eynde and Kutty, 2022). While applied to the diagnosis and treatment of multiple diseases, the utilization of AI particularly in AVSD detection is crucial due to the lack of efficient diagnoses by manual examinations. A 2020 study on the misdiagnosis of CHDs found that in 49% of missed cases, the lack of detection was due to poor adaptational skills resulting in inadequate images, in which the CHD was not visually recognized by the sonographers (Nisselrooij et al., 2020). AI techniques, including machine learning and deep learning, have therefore demonstrated promise when applied to fetal echocardiography.
Machine learning (ML) is a subset of AI that involves training algorithms to make successful predictions and/or decisions using past experiences. They can offer fast and accurate data due to the recent increase in storage capacity and processing power that are incomparable to those of humans. The primary distinction between AI and ML is the ability of ML to make its own decisions without being programmed to do so. If the expert system is able to scan or diagnose a patient without using parameters that were defined in its algorithm, it creates the possibility of detecting diseases or bodily structures that may not have been discovered or diagnosed before.
Deep learning is a subset of ML that consists of multiple layers. The greater the number of layers in the network, the greater the accuracy of the system in analyzing pictures, text and more. Deep learning uses methods that can be supervised, semi-supervised or unsupervised to be able to improve analytical tasks.
Efficient and accurate imaging tests for the prenatal diagnosis of AVSD are currently unknown and will require further research. Although it is known that the detection of AVSD can be improved through training programs and experience, the process requires significant amounts of time and investment. Due to the ability of AI, especially under supervised learning, to minimize human error, reduce bias, create a standardized system to decrease subjectivity between clinicians, and enhance performance in repetitive and consistent tasks, healthcare professionals continue to study the potential integration of AI to medical imaging (Day et al., 2021). Improved performance includes recognizing “correct echocardiographic view, and also [identifying] specific pathology within these views” (Day et al., 2021).
This review article aims to comprehensively explore the evolving role of AI in the prenatal detection of AVSD. By examining recent research and review papers, methodologies, findings, challenges and future directions, this paper provides an insight into the current understanding of AI, as well as its potential in the early detection of AVSD to reduce the number of patients developing the disease worldwide. This has the potential to transform the way AVSD is diagnosed and managed.
2. METHODS
The primary source for information and results used in this report were meta-analyses and review articles regarding the issue of early detection of AVSD using AI imaging. The results found were compared with each other and analyzed in order to address the matter of this report.
Selection Criteria
- Outcomes of the paper were in relation to prenatal detection as the paper is about the early detection of AVSD
- Sources were written by reputable individuals/groups e.g. Universities, well-established medical organizations (Nature Medicine, Obstetrics & Gynecology, etc.)
- Articles had a clear study design and structure
The sources used in this review article were found via research search engines such as PubMed and Google Scholar. When using these search engines and entering the keywords, the engine provides scholarly articles with topics pertaining to those keywords. For this review article, the keywords that were included were as follows: prenatal detection, AVSD, AI imaging in congenital heart disease. Sources used in this review article largely came from PubMed, as it is one of the largest online databases which contains thousands of scholarly reports and articles on a plethora of medical topics.
3. RESULTS
3.1. Imaging techniques
3.1.1. Echocardiography
The rapid advancements in diagnostic artificial intelligence in recent years have allowed for more accurate and efficient cardiovascular imaging techniques. More efficiency in this sort of technology provides access for “non-cardiologists” and use of AI in more regular clinical practice. AI software is being integrated into smaller point-of-care ultrasound devices to make swift assessments more accessible to non-cardiologists where necessary. Companies, such as Caption Health, have notably furthered this plan of access by developing a “deep-learning program” to ensure novice users can access standard echocardiographic images (Coulter and Campos, 2022). Software like that used by Caption Health decreases processing time and stress to the sonographer whilst optimizing image quality. Cardiac diagnoses rely on the quality of the echocardiographic images, which relies on the accuracy—and therefore experience—of the sonographer. However, when artificial intelligence can be programmed to optimize the images automatically, room for human error could be entirely eliminated. AI has the potential to commercialize fully optimized echocardiographic imaging, giving all patients access to swift and accurate diagnoses to begin appropriate care early.
3.1.2. Fetal Echocardiograms
Fetal echocardiogram is an ultrasound used for the prenatal diagnosis of AVSD. Day et al. (2021) emphasized that while fetal echocardiogram can antenatally detect CHD, the detection rates vary greatly, “with some countries detecting only 14% of severe CHD cases before birth”. Research was conducted with the fetal intelligent navigation echocardiography (FINE) method, an AI tool designed for the enhancement of prenatal AVSD detection. An original study by Yeo et al. (2018) examined the method of FINE to fetal echocardiograms. The study investigated the performance of FINE in a total of 150 fetuses, with 50 fetuses having CHD. According to the data collected, FINE has a sensitivity of 98%, specificity of 93%, and was able to accurately identify 74% of CHD cases (Yeo, Luewan and Romero, 2018).
In another study, by integrating the FINE method with assistance from a virtual intelligent sonographer (VIS), echocardiographic views of the fetal heart were generated and optimized; as a result, all AVSD diagnoses from the study were able to be identified (Veronese et al., 2023).
3.2. Early Detection and Prognostic Significance
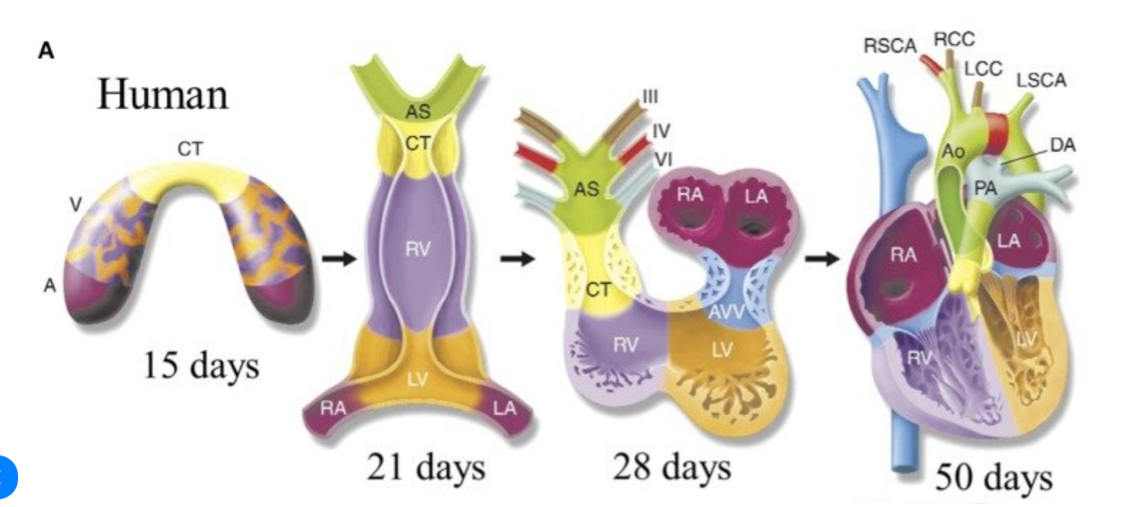
Figure 2. The stages of heart development. Illustrations depict cardiac development with color coding of morphologically related regions, seen from a ventral view.
A- atrium, V- ventricle, RV- right ventricle, LV- left ventricle, RA- right atrium, LA- left ventricle, CT- contruncal, AS- aortic sac, AVV – atrioventricular valve, PA- pulmonary artery, Ao- aorta, DA- ductus arteriosus, RSCA- right subclavian artery, LSCA- left subclavian artery, RCC- right common carotid, LCC- left common carotid (Butcher, 2014).
As seen in figure 2, the heart of the fetus undergoes four stages of development before birth. During these stages, due to the genomic variability in pregnancy trimesters , AVSD becomes more likely to develop (Jokhadze et al., 2021). This section, therefore, explores the importance of AI in the early detection of AVSD by understanding prenatal scans in each trimester.
3.2.1. AI in the First Trimester
The embryonic stage to the end of the first trimester lasts from the third week of pregnancy until the thirteenth week (Jukic et al., 2013). During this time period, a baby’s heart continues to develop. It is at this stage that the risk of a congenital heart disease becomes prevalent. Knowledge and regular observation of the gestational age plays a key role here. Despite variation being a common occurrence between pregnancies, cases involving shorter gestation periods may indicate more than just AVSD; extracardiac abnormalities accompany the atrioventricular septal defect. This is likely to result in higher mortality rates by restricting the growth of the fetus (He et al., 2021).
Nuchal translucency (NT) is a fluid-like sonographic region under the skin of the neck of the fetus. NT test is an optional, low-risk screening test taken in the first trimester. By measuring the amount of fluid behind the neck, calculations on the probability of having a genetic or chromosomal variant. According to He et al. (2021), “the NT measurement has proven to be a crucial parameter in prenatal screening”. However, although NT ultrasound is used to determine risk and provide recommendation for further medical tests, it is unable to diagnose a condition. If present with increased NT thickness, the fetus has a higher risk of CHD. When the NT thickness is above the 99th percentile of the usual measurement at the particular GA, a fetal echocardiography must be referred to. This differential scan will confirm which CHD has developed – including AVSD.
Transvaginal ultrasounds are commonly used for detailed images of the embryo. AI’s ability to detect intricate structures aid in the indication of fetal health. Moreover, it assists in confirming the GA and detects abnormalities.
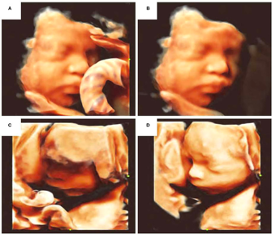
Figure 3. A comparison between regular 3D scans and AI-enhanced scans. A) Facial occlusion with umbilical cord B) Elimination of facial occlusion C) Facial edge incomplete reconstruction D) Facial imaging optimization (Chen, 2021).
Figure 3 demonstrates the ability of AI to detect and enhance fetal structures that are otherwise unclear to the sonographer- proving its capability to detect intricate structures, as well as enhance it in an older image. This will help obstetricians in advising the correct antenatal care required for both the progenitor and the fetus before and after a surgery. This is important as the after-effects of a neonatal surgery can last a lifetime.
From 2018 to 2021, a study was conducted with 4695 pregnant volunteers in North Carolina and Zambia to create an AI technique using deep learning for the calculation of GA. The advised AI model made use of deep learning – neural networks – to estimate the GA with accuracy similar to that of trained sonographers conducting standard fetal biometry (Pokaprakarn et al., 2022). Moreover, Van den Heuvel et al. engineered a way to determine the GA automatically from measurements made by AI in prenatal scans. He advised the use of a computer aided detection (CAD) system for automated measurement of the fetal head circumference (HC) in 2D ultrasound images for all trimesters of the pregnancy (Heuvel et al., 2018). The advantage of this solution is its ability to be used in the detection of AVSD. At different trimesters, there is a varying possibility of genomic variations and chromosomal microdeletion syndromes – a leading cause of CHD, including AVSD. Therefore, by confirming the GA, these causes can be looked for in fetal echocardiograms and other differential scans to detect AVSD before it is completely developed. However, it was also found in the same study that the likelihood of these abnormalities during pregnancy was similar to that of healthy non-pregnant women, thereby questioning its reliability.
Abdominal ultrasounds are also in use during pregnancy to monitor the health and development of the fetus as well as the progenitor. Here, AI enhances the analysis of biomarkers and structural abnormalities. Ultrasounds are noninvasive sonographs that use high-intensity waves to form real-time images of internal organs and other soft tissues – such as a fetus. Prenatal ultrasounds specifically are routinely used to check biomarkers of fetuses in the second trimester, such as the growth rate, amniotic fluid, and presence of congenital diseases. With the use of AI, however, diagnoses of severe or rare congenital heart diseases, such as AVSD, could be much more accessible. Recent AI developments would help eliminate human error when screening for antenatal disease.
3.2.2 AI in the Second and Third Trimesters
Sonographers evaluate fetal growth and dysplasia, the abnormal cell growth, with greater sensitivity in the mid-trimester. When second trimester scans are associated with third trimester checks, congenital abnormalities and postnatal outcomes can be better predicted (He et al., 2021).
In the mid-trimester, it becomes imperative to observe the progress displayed by the fetus’ vital structures, including the head and abdomen. AI tools are able to make examinations in the mid-trimesters more efficient. Examples of the implementation of AI include the automation of consistent and repetitive tasks and head circumference (HC) measurements (He et al., 2021). However, at this stage, scans become increasingly difficult to understand due to the inaccuracy made by the scan itself, which are affected by several factors such as abnormalities of the fetus and/or the scanning procedure, low contrast, and boundary occlusion. These factors tend to affect AI in detection as well as in the measurements of the scan’s outcome. The possibility of a 100% accurate scan being close to zero, and as a result, the accuracy of the machines in diagnoses decreases.
3.2.3 AI in 2D and 3D scans
The acquisition of accurate fetal images for obstetric examination is significant. Currently, most imaging methods are manual-based and therefore rely on an experienced operator with sufficient knowledge. One of the most important structures to examine in a prenatal ultrasound (US) scan is the fetal face. While conventional 2D US is the standard for such scans, it begs the question of whether AI endorsement would be more beneficial for 2D or 3D scans to detect and diagnose AVSD. While 3D scans are known to be more accurate than 2D scans, both without the use of AI, a 2019 study on the difference between 2D and 3D scanning catered to AV-valve imaging showed that both image quality and accuracy were similar (Hakacova et al., 2019). The test involved 20 children (mean age: 8 months) with AVSD. In each patient, 26 AV-valve features were involved in the assessment.
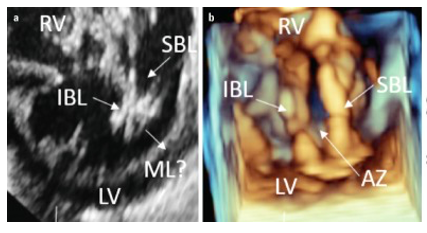
Figure 4. Comparison of 2D and 3D echocardiography of the AV-valve. a) 2D echocardiography of the AV-valve b) 3D echocardiography of the AC-valve. b) LV – left ventricle; RV – right ventricle; IBL – inferior bridging leaflet; SBL – superior bridging leaflet (Cardiol, 2019).
Figure 4 provides an example of when 3D echocardiography disagreed when assessing the valve erosions. 2D echocardiography shows the presence of erosions in the superior and inferior bridging leaflet. The research concluded that in almost 48% of cases where the 2D was accurate, the 3D was inaccurate, and vice versa. AI can assist in the correction of this issue. AI can be utilized to assess scan quality as was recently established in hip ultrasounds (Hareendranathan et al., 2021). Using expert systems, AI assessment of the quality of a medical scan can provide solutions to improving both 2D and 3D scanning accuracy, which can be implemented in early AVSD detection.
3.2.4 AI in the diagnosis of AVSD
Expert systems are a form of AI that were developed to mimic human knowledge and experiences – they use knowledge and inference to solve problems or answer questions that would otherwise require a human expert (Torasso, 1985). These systems output a conclusion and how it was reached, along with the percentage probability of the accuracy of its conclusion.
Expert systems consist of a user interface, explanation system, inference engine, rules base and knowledge base. The user interface is the method by which the system interacts with the user using command prompts or other input methods. The inference engine asks a series of questions based on the user’s responses to gather relevant information – it acts like a search engine by examining the knowledge base for information that matches the user’s query. Finally, the rules base contains the set of inference rules used to draw conclusions.
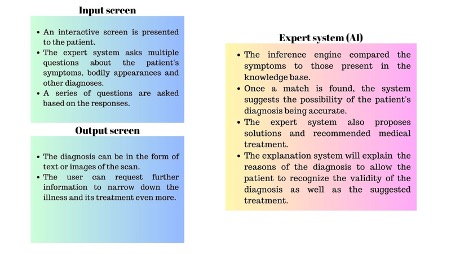
Figure 5. An example of how an expert system is used to perform a medical diagnosis.
In order to help physicians to make a better and faster diagnosis of diseases in particular tropical diseases, an expert system was made called MITSABO. MITSABO’s development was divided into three parts. In the first one, they showed the AI the different steps of a physician’s diagnosis of a patient. The second part is related to the architecture of the expert system, including the application of the method of data analysis. The results thus obtained are made more precise by the application of the inference engine, which itself uses the knowledge base system. In the third part the clinical conclusion is provided (Raboanary et al., 1995). However, it is unknown whether the MITSABO system was discontinued.
The use of such an expert system would be extremely beneficial if applied to automatic diagnosis of AVSD. This means that after a prenatal scan, an expert system could immediately diagnose AVSD as well as other abnormalities by observing the structures present in the image; presenting a percentage probability of developing the disease or its concerned symptoms. It may propose prenatal care as medical treatment, for AVSD can still be caused by certain environmental factors, which the expert system can try to identify using the defined parameters- similar to machine learning. Moreover, since the exact causes of AVSD remain unknown, machine learning can aid the identification of said causes.
DISCUSSION
There are already a plethora of methods in which AI imaging can be utilized in the early detection of AVSD. AI is used in the first trimester, when the risk of developing AVSD first presents and hence why it is crucial that any signs are detected as soon as possible. This is done possible through transvaginal ultrasounds, which provide detailed pictures of the embryo and AI can then predict gestational age and identify any abnormalities. AI can perform these actions to a much higher degree of accuracy. If these procedures are performed manually, the results could have some form of human error, skewing the reliability of the results.
The second and third trimesters are periods when more accurate predictions on the embryo’s heart can be made with its further development. More defined vital structures, such as the head and the abdomen, become crucial in the detection of AVSD as well. However, the major issue in this stage is that since the organs of the embryo have become more developed, reading and analyzing the scans can become more complicated for both humans and AI. Aside from this issue, the more information and details doctors can receive regarding the embryo allows for the prediction of postnatal outcomes.
Echocardiography is one of the many forms of imaging that has resulted from further advancements in AI and technology, which allows us to use these techniques to produce more accurate assessments on the presence of cardiovascular diseases. More efficient imaging techniques in cardiology allows more users to access these technologies with less formal training and to use them in more regular clinics, with AI assisting these individuals in understanding echocardiographic images. With further progress in the development and advancement of cardiovascular imaging techniques, they can then be accessible to all patients early, allowing for possibly sooner diagnosis and therefore treatment of cardiovascular diseases like AVSD.
AVSD is only one of many congenital heart defects, however its early detection is one of the most crucial. Since the causes of AVSD are still unknown, one must rely on its early detection to allow for earlier diagnosis and subsequent treatment, which in turn can possibly lower the mortality rate associated with AVSD. If the defects were not to be detected, then the consequences could be fatal. Left atrioventricular valve regurgitation, residual intracardiac shunts and various life-threatening arrhythmias could all arise if AVSD is not detected and thus treated early (Ahmed and Anjum, 2023). With earlier detection, children diagnosed with AVSD can also receive the necessary operations, though at varying stages depending on the severity.
However, some concerns arise from the commercialisation of AI imaging in cardiology, and in the medical industry at large. The commercialisation may make the technology more accessible and possibly usable by non-specialised individuals, such as clinics and GPs, although, this may impact the quality. Companies may value making a profit over the quality of a product, which in turn can be problematic, especially in a medical field. Despite this, as of now, the continuous development and advancements made in cardiovascular imaging technology and its widespread use for non-cardiologists will be beneficial to the diagnosis and treatment of AVSD.
Questions pertaining to a particular disease asked to expert systems progressively increase; the variation between right and wrong answers will lead to increased misdiagnoses and false treatment plans. It may also cause a lack of difference between medical expert systems and those created by corporations looking for increased profit. Furthermore, ethical concerns on the use of AI continue to limit the widespread use of AI in the medical field, namely data privacy of patients and various other privacy complications – particularly because AI requires personal data in order to make inferences and conclusions, especially for unsupervised learning and similar AI techniques.
CONCLUSION
AI imaging is effective when used in the early detection of AVSD. Both machine learning and deep learning have revolutionized medicine due to the increased accuracy and specificity of prenatal scans, particularly fetal echocardiography. Supervised learning, the subset of machine learning, is the primary AI technique utilized in these scans. The use of predefined datasets enables obstetricians, gynecologists and perinatologists to improve patient care as the scans can now be conducted by AI. Although further research and assessments are required, AI imaging holds great potential in detecting the AVSD before its symptoms develop, or even before the genetic deletion takes place. However, as this technology becomes more powerful and widely used, it becomes less transparent. Studying AI and AVSD closely could lead to self-diagnoses of the disease, creating opportunity for patients to detect and diagnose themselves in other CHD as well.
Bibliography
Ahmed, I. and Anjum, F. (2023). Atrioventricular Septal Defect. Available at: https://europepmc.org/article/NBK/nbk562194 (Accessed: 19 August 2023).
Arafati, A., Hu, P., Finn, J.P., Rickers, C., Cheng, A.L., Jafarkhani, H. and Kheradvar, A. (2019). Artificial intelligence in pediatric and adult congenital cardiac MRI: an unmet clinical need. (Accessed: 20 August 2023).
Boston Children’s Hospital What is atrioventricular canal defect? Available at: https://www.childrenshospital.org/conditions/atrioventricular-canal-defect (Accessed: 25 August 2023).
Centers for Disease Control and Prevention. (2023). Atrioventricular Septal Defect. Available at: https://www.cdc.gov/ncbddd/heartdefects/avsd.html (Accessed: 19 August 2023).
Chen, Z., Liu, Z., Du, M. and Wang Z. (2021). Artificial Intelligence in Obstetric Ultrasound: An Update and Future Applications. Available at: https://www.ncbi.nlm.nih.gov/pmc/articles/PMC8429607/ (Accessed: 24 August 2023).
Cleveland Clinic. (2022). Nuchal Translucency. Available at: https://my.clevelandclinic.org/health/diagnostics/23333-nuchal-translucency (Accessed: 26 August 2023).
Cleveland Clinic. (2022). Ultrasound. Available at: https://my.clevelandclinic.org/health/diagnostics/4995-ultrasound (Accessed 26 August 2023)
Coulter, S.A. and Campos, K. (2022). Artificial Intelligence in Echocardiography. Available at: https://www.ncbi.nlm.nih.gov/pmc/articles/PMC9053659/ (Accessed: 19 August 2023).
Craig, B. (2006). Atrioventricular septal defect: from fetus to adult. Available at: https://www.ncbi.nlm.nih.gov/pmc/articles/PMC1861295/ (Accessed: 19 August 2023).
Day, T.G., Kainz, B., Hajnal, J., Razavi, R. and Simpson J.M. (2021). Artificial intelligence, fetal echocardiography, and congenital heart disease. Available at: https://www.ncbi.nlm.nih.gov/pmc/articles/PMC8641383/ (Accessed: 20 August 2023).
Dey, D., Slomka, P.J., Leeson, P., Comaniciu, D., Shrestha, S., Sengupta, P.P. and Marwick, T.H. (2019). Artificial Intelligence in Cardiovascular Imaging. Available at: https://www.ncbi.nlm.nih.gov/pmc/articles/PMC6474254/ (Accessed: 19 August 2023).
Fornell, D. (2023). Cardiology has embraced AI more than most other specialties. Available at: https://cardiovascularbusiness.com/topics/artificial-intelligence/cardiology-has-embraced-ai-more-most-other-specialties (Accessed: 20 August 2023).
He, F., Wang, Y., Xiu, Y., Zhang, Y. and Chen, L. (2021). Artificial Intelligence in Prenatal Ultrasound Diagnosis. Available at: https://www.frontiersin.org/articles/10.3389/fmed.2021.729978/full (Accessed: 20 August 2023).
Lim, L.J., Tison, G.H. and Delling F.N. (2020). Artificial Intelligence in Cardiovascular Imaging. Available at: https://www.ncbi.nlm.nih.gov/pmc/articles/PMC7350824/ (Accessed: 20 August 2023).
Lindsey, S.E., Butcher, J.T. and Yalcin, H.C. (2014). Mechanical Regulation of Cardiac Development. Available at: https://www.researchgate.net/publication/265393829_Mechanical_Regulation_of_Cardiac_Development (Accessed: 26 August 2023).
Reddy, C.D., Eynde, J.V.D. and Kutty, S. (2022). Artificial intelligence in perinatal diagnosis and management of congenital heart disease. Available at: https://pubmed.ncbi.nlm.nih.gov/35396036/ (Accessed: 19 August 2023).
Sermesant, M., Delingette H., Cochet, H., Jaïs, P. and Ayache, N. (2021) Applications of artificial intelligence in cardiovascular imaging. Available at: https://www.nature.com/articles/s41569-021-00527-2 (Accessed: 20 August 2023).
Siontis, K.C., Noseworthy, P.A., Attia, Z.I. and Friedman P.A. (2021) Artificial intelligence-enhanced electrocardiography in cardiovascular disease management. Available at: https://www.nature.com/articles/s41569-020-00503-2 (Accessed: 20 August 2023).
Słodki, M., Soroka, M., Rizzo, G., Respondek-Liberska, M. and International Prenatal Cardiology Collaboration Group. (2020). Prenatal Atrioventricular Septal Defect (AVSD) as a planned congenital heart disease with different outcome depending on the presence of the coexisting extracardiac abnormalities (ECA) and/or malformations (ECM). Available at: https://pubmed.ncbi.nlm.nih.gov/30514144/ (Accessed: 24 August 2023).
Van Nisselrooij, A.E.L., Teunissen, A.K.K., Clur, S.A., Rozendaal, L., Pajkrt, E., Linskens, I.H., Rammeloo, L., van Lith, J.M.M., Blom, N.A. and Haak, M.C. (2020). Why are congenital heart defects being missed? Available at: https://pubmed.ncbi.nlm.nih.gov/31131945/ (Accessed: 24 August 2023).
Veronese, P., Guariento, A., Cattapan, C., Fedrigo, M., Gervasi, M.T., Angelini, A., Riva, A. and Vida, V. (2023). Prenatal Diagnosis and Fetopsy Validation of Complete Atrioventricular Septal Defects Using the Fetal Intelligent Navigation Echocardiography Method. Available at: https://pubmed.ncbi.nlm.nih.gov/36766561/ (Accessed: 19 August 2023).
Yang, H., Pan, J., Wang, W., Guo, T. and Ma, T. (2023). Application of Artificial Intelligence-Based Auxiliary Diagnosis in Congenital Heart Disease Screening. Available at: https://www.ncbi.nlm.nih.gov/pmc/articles/PMC10098384/. (Accessed: 20 August 2023).
Yeo, L., Leuwan, S. and Romero, R. (2018). ‘Fetal Intelligent Navigation Echocardiography (FINE) Detects 98% of Congenital Heart Disease’, Journal of Ultrasound in Medicine, 37(11), pp. 2577-2593. Available at: https://doi.org/10.1002/jum.14616 (Accessed: 24 August 2023).