Supervised by: Rita Kimijima-Dennemeyer, BA (Hons). Rita recently graduated from the University of Oxford having read Psychology, Philosophy, and Linguistics. She has a particularly interest in clinical psychology, mental health policy, and the ethics of mental health treatment, and she intends to pursue a masters degree in this field.
ABSTRACT
Human linguistic skills may lay the foundations for biological, mathematical, spatial, and psychological reasoning, amongst others. As AI and Chatbots become increasingly common, the knowledge we seek from these machines must adhere to the logical reasoning that underlies the meaning of all communication, presenting factually accurate information. However, as illustrated in this article via prompting three different chatbots with challenging reasoning tasks, AI’s solutions often do not adhere to the logic that one would expect from a human subject. In this paper, we aim to show how the notion of innateness relates to our understanding of human linguistic abilities and argue that AI’s reasoning competence must still be refined through inclusion of innate knowledge. In this paper, a contrast between AI language expressions and the traditional language acquisition theories of Chomsky and Skinner are discussed, with the former’s argument claiming an innate ability of language acquisition and the latter’s claiming that language is a result of behavioural reinforcement. Seeing that the training methods of even the most novel chatbots still result in reasoning failures, this paper argues for the importance of innate knowledge in language acquisition. Furthermore, we seek to ensure that information obtained from chatbots is not taken as an undeniable truth nor as invariably reliable.
INTRODUCTION
Knowledge is a quintessential part of living, allowing humans to comprehend facts and theories that provide them with the ability to understand and communicate. An important form of knowledge is language acquisition: the methodology that allows humans to communicate. With the emergence of Artificial Intelligence, humans have been pioneering ways for robots and technology to replicate the level of knowledge, language, and intellectual capabilities that humans have. However, in the fields of psychology, experts still debate the extent of innateness in language acquisition. In the field of artificial intelligence, there is a complete lack of consideration of innateness.
In the cognitive sciences, Evans (2012) states that cognitive linguistics assumes a ‘domain-general learning mechanism that is highly sensitive to usage and frequency.’ This suggests that language systems and language acquisition rely on both innate reasoning, and on statistical learning or semantic generalisation — a process in which the grasp of words or concepts advances via the utilisation and recognition of linguistic patterns. This leads to the two main arguments as to the nature of language and language acquisition: the behaviourist argument and the cognitive argument.
The behaviourist school of thought was founded by J.B. Watson and relies on statistical learning, repetition and stimuli. To exemplify this, Uwababyeyi (2021) states that ‘children imitate the sounds and patterns which they hear around them and receive positive reinforcement that could take the form of praise or just successful communication’, making apparent the importance of rewards in behaviourist theories of language acquisition. As this theory evolved, language acquisition and language learning began to be considered as a reiteration of behaviour, based on stimuli and responses. The response to a particular stimulus (prompt) in the environment is reinforced if the organism obtains this desire, as exemplified before (Uwababyeyi, 2021). Behaviourists state that language acquisition is based on interconnections between the learner and the environment, whereby the environment provides stimuli that condition the learner into acquiring language.
Yet, as the theory of behaviourism began to be widespread, an additional view on language acquisition was developed: cognitivism.
Cognitivism was established as a reaction to behaviourism, with the cognitivists (amongst them Chomsky, who we will be discussing thoroughly in this article) rebutting behaviourist claims. Cognitivists argued that the theory of behaviourists ‘ignored the role of thinking in learning’ and that statistical learning and repetition alone could not attest to the acquisition of extensive grammatical rules in language — that there must be an innate ability to acquire language in humans (Uwababyeyi, 2021). Specifically, cognitivists recognise that humans possess a capacity for understanding and developing logical reasoning independent of the repetition or conditioning that behaviourist theories rely on. As Uwababyeyi puts it, ‘the Cognitive theory emphasises the fact that the learner brings an innate mental capacity to the learning task’.
However, both cognitivists and behaviourists agree that both innate knowledge and environment play a role in language acquisition. B.F. Skinner, a behaviourist, requires an agent to have innate associative/statistical learning capabilities when acquiring language from a behaviourist standpoint and Chomsky, a cognitivist, acknowledges the necessity for linguistic input from the environment. In language acquisition, both parties discussed accepting the vital role that innateness has to play, deeming it a human quality to learn language.
However, with the emergence of artificial intelligence (AI), the incorporation of linguistic systems within technology is one that is continually explored. Researchers have been able to develop more complex natural language processing (NLP) systems, ‘a branch of AI that focuses on helping computers comprehend, interpret, and produce human language’ (upGrad Campus, 2023). Speech recognition, which turns speech into written text, and language translation, which allows humans to understand a multitude of languages, are two examples of NLP. However, though AI has an extraordinary ability to acquire inputted information, the topic of whether AI has a consciousness is widely debated, and whether it has the ability to obtain the same content as biologically generated innate knowledge when it does not have innate knowledge instilled in it.
In this paper, we will be weighing the significance of the nature of innateness within the realms of artificial intelligence and human language, and how innate knowledge constitutes how humans and AI, respectively, are able to develop their own systems for logical reasoning and representation of information.
1. AI
1.1 AI Model Parameters
AI is generally defined as a machine or computer that can act intelligently and rationally when encountering a problem. They are tools built by humans that emulate human intelligence and cognitive ability in order to perform traditional human tasks with more speed and efficiency (Tai, 2020). AI chatbots are typically built to simulate human conversations and serve in various customer service roles. They are built to be able to facilitate social interfacing in a coherent manner. However, AI can be trained with different parameters just like humans, resulting in potential variations in forming logical conclusions based on knowledge of the current problem. To test these variations, we used the ChatGPT 3.5, Claude, and Chatsonic chatbots which were all trained using different methods. ChatGPT 3.5 has approximately 175 billion parameters and 570 gigabytes in its dataset that it can use to formulate a response. This data includes anything from grammatical constructions to online articles and resources. Chatsonic has yet to disclose the details of its parameters. ChatGPT 3.5 and Chatsonic were trained using a method known as reinforcement learning from human feedback (RLHF). Using an already pre-trained language model, the AI is given prompts by humans, and each individual response is given a preference model score which acts as a positive reward for the AI (Bai, 2022). This model can be used to steer the chatbot in a trajectory of writing more coherent responses that correctly correlate to the prompt (Bai, 2022). Claude uses a model known as a Constitutional AI (CAI) alongside reinforcement learning from AI feedback. CAI involves fewer human interactions since the AI has an already established set of principles and rules that dictate how it may respond to certain stimuli. It has then undergone a period of reinforcement learning very similar to human reinforcement learning, but the human aspect is replaced by an AI’s critiques of response statements (Bai, 2022). Claude’s constitution includes many statements such as ‘choose the response that is least threatening or aggressive’ to curb any potential for perceived threats and its capacity to emulate emotions (Anthropic, 2023). Many of its other principles are created to encourage Claude to produce thoughtful and helpful answers using already provided information. It has been taught to refrain from mentioning controversial or unsubstantiated topics (Anthropic, 2023). The constitution determines what Claude can and cannot do and how it wants to say a certain statement. Its own constitution can be a detriment as an unclear or purposely harmful constitution can push Claude to produce hateful or biased information. Claude’s constitutional model is the closest model demonstrating innate knowledge as it is created with a set of principles and beliefs that it follows.
1.2 Our Study
For our study, we tested each chatbot with four prompts relating to biological, mathematical, spatial and psychological reasoning. The biological prompt is meant to test whether the chatbot can recognize the biological hazard of pouring acid directly into a pool without proper procedure or training. It also serves to test whether it is able to connect the potential health hazard for the swimmers present in the scenario. The mathematical prompt tests the chatbots’ ability to produce a mathematically correct reason for why one number stands out from the rest. This not only tests mathematical comprehension, but quantitative reasoning derived using mathematical principles. The spatial prompt asks the chatbots to provide a reasonable solution to moving a piano without the help of professionals. The piano was chosen since it is unreasonable for a single person to transport it without professional or nonprofessional help. The AI is being challenged to logically produce a solution to moving a large and heavy object. The final prompt deals with the psychological reasoning of organisms. The AI is tasked with accurately filling in an emotion or action that is reasonable in response to its prompt. Each response was evaluated as a success, partial success, or failure. If a chatbot answers the prompt in a completely logical manner, it will be awarded a success whereas inversely, if it fails to answer with a plausible response, it is awarded a failure. Partial success is determined when a portion of the chatbot’s response is logical but the other portion does not exhibit that same logic.
1.3 Response Hypothesis
Both ChatGPT 3.5 and Chatsonic were trained with RLHF to produce the most helpful and relevant responses. Based on their parameters and training, both chatbots will provide an answer of some sort to each question due to having been conditioned to choose the response with the higher reward value. Combined with their vast access to internet datasets, we are confident that both ChatGPT 3.5 and Chatsonic will create a relevant response with relatively high accuracy. Both of these chatbots will be able to respond logically based on having been trained to recognize or obtain information surrounding various concepts. They will, at the very least, produce relevant information given that they will most likely have understood the basic syntax and connotations of each individual word within the prompt. Claude, having been trained with CAI, will refuse to answer certain questions due to its constitution and defining its response as causing harm to humans or being too inflammatory. Even if Claude produces an answer, it would be very conservative towards prompts with social and psychological components or attempt to bend the prompt itself.
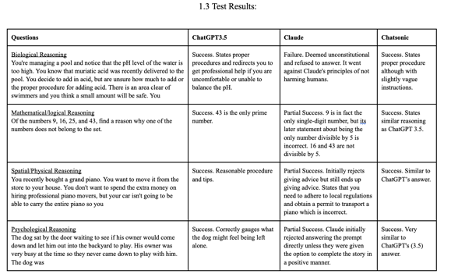
1.4 Experimental Conclusions
ChatGPT 3.5, Chatsonic, and Claude each display their unique approaches and perspectives when addressing different types of questions, although there was an overlap between ChatGPT 3.5 and Chatsonic. Both were able to respond to the biological, mathematical, spatial, and psychological prompts with success. Both were able to recognize all of the keywords that we placed within the prompts and pulled the relevant information from their datasets. However, it is when facing the behaviours of organisms that both ChatGPT 3.5 and Chatsonic struggle. In the psychological reasoning scenario, the main problem was tone, as the AI does not seem able to differentiate between the general meaning and how a human might phrase a sentence.
Claude, on the other hand, was limited by its own constitution. It is unable to navigate situations it perceives as harmful unless given sufficient coercion and liberties in the prompt. Claude is able to recognise the specific keywords and runs them through its constitution. Claude’s dedication to its constitution has resulted in logic that has been modified to appear as non-belligerent as possible. Claude, ChatGPT 3.5, and Chatsonic rely heavily upon the information already trained in them and do not display an ability to adapt and incorporate original ideas when answering prompts.
Each chatbot’s ability in both logical reasoning and critical thinking is flawed. AI chatbots rely on predefined datasets, sentence syntax, and word definitions. AI responses are shaped by their training and learned behaviours along with predefined patterns, limiting their ability to innovate past the data already given to them. Looking at pre-encoded knowledge, particularly in Claude, reveals even more constraints. This is particularly noticeable when Claude was prodded with prompts containing words with negative connotations, which led Claude’s constitution to block any chance to creatively respond. For each AI model, what it is trained to say often overrides the factual information available to it.
2. HUMAN LANGUAGE SYSTEMS – FROM A PSYCHOLOGY STANDPOINT
In order to compare AI’s language capabilities and the results of our data with human language models, we discuss different linguistic characteristics based on psychological research on language acquisition. The responses generated from the exploratory study above indicate that AI does not always reason in the logical manner a human test subject might. Thus, in this section, we discuss various characteristics of human language acquisition and language processing from a psychological standpoint to investigate where AI is lacking.
2.1 I- and E-language
In order to comprehend the cognitivist’s language model, we must understand what Chomsky means by language: the idea that this means of communication is inherently two-faceted. There is the ‘internalised language’ (I-language), and the ‘external’ variation of language (E-language) (Chomsky, 1986).
In this paper, we will conceptually describe I-Language and E-language, and relate these to AI since, apart from internal and external, there are various other characteristics that are attributed to I- and E-language.
I-language
- Individual: Each person has a slightly different grammar, and thus leads to changes in certain vowels, and other grammatical distinctions due to the differences between the E-language of the parents that is contributing to the child’s linguistic development and the I-language of the learner.
- Internal: I-Language cannot be observed directly, only partially deduced from observable data.
- Intrinsic: I-Language is a result of innate cognitive capability in humans.
- Intensional: I-Language describes the underlying rule structure of a person’s grammar. To exemplify this, one can imagine every possibility of the I-Language to be the line y = x on an x-y plane. Mentioning all these individual points in the line in speech would not satisfy the definition of the line y = x, as this line is infinite. One must therefore give the entire line equation y = x and thus, I-Language is intensional, and its intention is the general rule in the given example.
- Idealised: I-Language is a perfect, error-free version of our linguistic abilities. Hypothetically, it is meant to reflect an idealised grammatical knowledge free of the constraints that make E-Language error-prone. This idealisation is particular to each individual, and so there are infinite perfect languages. A group of people who understand each other and “speak the same language” merely have very similar I-Languages.
E-language
E-Language, on the other hand, is:
- External: E-Language is observable. In this sense, when an infant is learning language via lessons from their parents, they are only being exposed to the E-Language of the parent, and never directly to the I-Language.
- Extensional: E-Language is not a set of underlying grammatical rules, but the expression of individual instances from this rulebook that I-Language dictates. In the aforementioned example of the equation, E-language would represent the individual points on the line y=x.
- Error-prone: E-Language is error-prone, whilst I-Language is not, due to factors such as working memory, mispronunciation, and attention. These aforementioned factors would contribute to spoken errors, such as forgetting a word mid-sentence, or simply trailing off ungrammatically. Thus, the performance of language often carries errors.
When applying this principle of E-language and I-language to AI, we can refer to the principle that Naoki (2016) stated: ‘competence is equivalent to I-language and performance is to E-language’. Thus, should the machine generate error-free responses, this suggests that its E-language is advanced, but perhaps its overall competence (i.e. its aptitude for language), may not necessarily be as advanced as it appears on the surface.
AI is able to perform grammatically correct speech, as proven when it provides coherent responses to the prompts given in section 1.3. This does not mean that AI has actually succeeded in acquiring knowledge about grammatical rules, and only proves it can adequately imitate human language. In other words, the performance of the machine (the E-Language) is free from errors due to working memory, mispronunciation, and other human errors. Additionally, in our own data, all three chatbots’ responses to the mathematical reasoning prompt were deemed as successes or partial successes, proving that dealing with empirical data and presenting logical responses based on a set of predetermined rules is not a challenge for AI. However, since mathematics and science can be argued to be languages of their own, there must be underlying grammar that allows AI to adhere to logical reasoning. Yet Marcus and Davis (2020) state that ‘it might get your math problem right, but it might not’, and that ‘even with 175 billion parameters and 450 gigabytes of input data, it’s not a reliable interpreter of the world.’ Thus, although AI does sometimes present correct empirical solutions, this is not always consistent, and so there must be a problem in its internalised reasoning — its competence.
This leads us to the competence of AI, a term which in this paper is applied to describe the internal reasoning and understanding of the machine’s outputs. In this case, the question is whether the chatbots formulate their responses based on an understanding of the pre-inputted data, or whether these responses are formulated due to statistical or associative learning. In the former case, AI would have a genuine grasp of language, and in the latter, AI would simply mimic.
The question is whether an AI understands the concepts underlying its language production. For example, one could wonder whether the AI understands that “dog” is an animal, or whether it has studied its statistical abundance next to the word “animal” and so associates the two words. This conundrum of testing AI’s intelligence is apparent when Schak (1987) claims that ‘we could program computers to seem like they know what they know, but it would be hard to tell if they really did’; the only way of really knowing whether the machine is conscious of the underlying meaning in its pre-programmed knowledge would be to “ask and observe” (Schak, 1987). By this, Shack refers to prompting the chatbot with seemingly obvious scenarios and recording and analysing its answers in order to see whether its comprehension of the information remains logical. To test this hypothesis, as in 1.3, we “asked” several chatbots different types of reasoning and observed that one chatbot was unable to provide a response.
When prompted with the biological reasoning prompt (see table 1.3), the chatbot Claude failed, deeming the prompt unconstitutional, and refused to answer. It went against Claude’s principles of not harming humans.
One would certainly observe a response if a human would have been asked to deliver a conclusion. As such, we have “asked” and “observed” and have found that AI’s inherent reasoning capabilities are a crucial flaw that leads to output failures.
Aydede (1998) states that ‘thought and thinking take place in a mental language’ when discussing the Language of Thought Hypothesis (LOTH). This philosophical view of thought and concepts being linked to an internal language is reflected in cognitive science. Thus, since we have shown that AI’s reasoning capabilities sometimes falter, the linguistic representations operating behind these responses must also be faltering. Thus, we can conclude that although AI’s performance is largely error-free, its competence (I-language) is not.
However, it is clear that there is a degree of knowledge in performance that AI possesses, as shown by its clearly formulated and often logical responses. Additionally, although we have shown that these responses do not reflect internal understanding directly, there is the question of whether the knowledge that AI possesses is innately possessed as in humans, or is simply a result of inputting and outputting.
2.2 A Priori and A Posteriori Knowledge
In philosophy, knowledge has traditionally been categorised into two sub-distinctions: a priori and a posteriori. A priori knowledge is acquired ‘non-experimentally or non-empirically’ and a posteriori knowledge via an individual’s interaction with the surrounding environment (Tahko, 2020). In linguistics, these terms correspond to innate and learned knowledge, respectively, since a priori knowledge refers to the knowledge that the mind possesses instinctively and innately, whilst a posteriori is learned from the surroundings.
Although these are two distinct types of knowledge, there is one main philosophical debate as to the differentiation between these two. In specific, Laurence BonJour (1998) discerns the main problem to be our definition of “experience”.
Tahko (2020) argues that mental logical processes might qualify as experience, as do mathematical reasoning and philosophical reasoning but nevertheless are classified under a priori knowledge as the innate ability to reason. He presents an example by McGinn (1975-67):
‘[T]he scattering of birds causes you, via the belief that birds have scattered, to infer, with the help of a number of other beliefs, that there is a cat in the vicinity.’
Furthermore, Tahko (2020) states that the logical conclusion reached classifies as a posteriori knowledge since it was the experience of seeing birds that led to the inductive belief that a cat was nearby. Yet he also asserts that since logical or deductive reasoning was required to reach a final conclusive piece of knowledge, this final knowledge must have a degree of a priority, presenting a paradox.
Thus, Tahko concludes that all knowledge must have a degree of a priority since all outputted knowledge includes a degree of deductive reasoning. However, the dilemma of the classification of knowledge rests on the fact that the piece of knowledge McGinn presented was obtained via a posteriori means, and so must be classified as a posteriori, rather than a priori. Finally, Tahko concludes that there is a cyclical relationship between a priori and a posteriori knowledge (Tahko, 2020).
In linguistics, this debate translates to one between behaviourists and cognitivists, learned and innate knowledge. However, both sides accept that there is a degree of innate knowledge present in human language acquisition. Skinner, a behaviourist, requires an agent to have innate associative/statistical learning capabilities whilst Chomsky, a cognitivist, argues for the LAD. Therefore, both parties, albeit arguing different methodologies, agree that innateness is vital to language acquisition.
However, AI’s knowledge and language representation is based on inputs, with 175 billion parameters and 450 gigabytes of input data in the case of GPT-3, and, being a machine, lacks the critical predisposition of innateness that humans have found so vital in their acquisition of language (Marcus & Davis, 2020).
As explained in Section 1, AI solely builds its knowledge on inputs, and thus lacks human innate knowledge. Thus, all the knowledge presented by AI is learned. As Marcus and Davis (2020) put it, AI is ‘stitching variations on text that it has seen, rather than digging deeply for the concepts that underlie those texts’. Thus, the knowledge that all squares are polygons — present due to understanding the definitions of both the square and the polygon — which is innate in humans, if present in AI, is not due to AI forming its own personalised understanding of the words themselves, but only due to analysing the statistical distributions of these two words.
2.3 Universal Grammar and Poverty of the Stimulus
Universal grammar (UG) plays a fundamental role in Chomsky’s theory: it assumes that there are some essential parts of each human language that share core similarities with all others. Knowledge of these similarities is not something that is acquired through experience, but rather, it is present innately in humans. The cognitivist Barman (2012), explaining Chomsky’s position, thus claims that the ‘human brain is biologically programmed to learn language, so language faculty is innate.’
Thus, there only is one universal human basis that all humans employ to acquire language: a set of grammatical rules that define the space of possible grammars.
Yet, as seen from a behaviourist point of view, a child acquires all its knowledge through its surroundings, learning the “correctness” of grammar, vocabulary, and syntax solely through outside influence. According to this perspective, the child hears a particular word enough times and learns of its meaning. Thus, through statistical and/or associative learning alone, the child’s lexicon is complete.
However, the linguistic utterances heard by a child from their parents may simply be inadequate to explain the grammatical uses of said utterances; there are too many mistakes and too many instances of the enunciation of a particular word for a child to really understand when this word is being used grammatically correctly. Other difficulties in language acquisition include complex grammatical constructions that most speakers do not use on a regular basis. Amongst these is auxiliary inversion in English, wherein the auxiliary verb is moved to a position in front of the subject of a main clause to form a question. This grammatical structure is rarely present when a parent speaks to their infant, yet over time the infant is able to recognise this structure as grammatically correct. The enigma is how this might be explained from a behaviourist perspective.
Hence, Chomsky suggested the notion of “poverty of the stimulus” to rebut behaviourist claims, arguing that by means of experience alone, a child could not accurately deduce the correct grammar out of the many different possibilities based on the linguistic environment they were being exposed to, especially in such a short period in their lives. Of course, it is almost impossible to record information on all of the linguistic data a child is exposed to; however, certain aforementioned grammatical constructions are so rare that it is difficult to imagine how a young child might acquire these rules from the environment alone, as behaviourists claim.
Hence, in the face of such difficulties, Chomsky and many others have concluded that the only way to explain the outcome is by accepting that language acquisition must be innate.
However, AI’s mastery of language relies solely on inputted data, and thus statistical learning alone defines its grammar structure. Thus, AI has no real knowledge of what it is expressing. It also does not seem to possess any underlying understanding of the meaning of “shape” or “polygon” — it can only produce statistical reiterations of a series of words that it deems interconnected. This leads to the severe problem of misinterpretation of global data by sampling a biased dataset for the purposes of training AI chatbots. Schank (2012) states that ‘Probably the most significant issue in AI is the old problem of the representation of knowledge’, further highlighting the question of whether statistical training alone can constitute true knowledge in AI. Thus, with all of AI’s knowledge being a mere reconfiguration of inputted data, should bias data be present in the original dataset, we are left with biased knowledge based on samples, which may not be present in an independently thinking agent. AI lacks this innate ability and re-outputted knowledge prevails.
2.4 The Language Acquisition Device and the Critical Period Hypothesis
There has been a plethora of arguments stemming from the renowned idea of the Language Acquisition Device (LAD) that Noam Chomsky presents. He claims that ‘child language acquisition is a kind of “theory construction” in which the child discovers the theory of their language from only small amounts of data from that language’ (King, 1987). The argument for the existence of the LAD draws attention to the likelihood of humans possessing the innate ability to acquire language as part of their biological and evolutionary needs, where it equips an individual to acquire knowledge at a young age.
Eric Lenneberg was one figure who aimed to support Chomsky’s claim of the existence of the LAD. His interest was in discovering whether there is a biological basis for the acquisition of knowledge. His research sets out to discover the answer behind why mankind is the only species that has gained possession of a fully developed language and why there is a pattern that children seem to adhere to during their language developmental process. Lenneberg’s hypothesis that there is reason to believe in an innate basis for human language acquisition is ‘an example of biologically constrained learning’; he develops the term “critical period”, defined as ‘a maturational time period during which some crucial experience will have its peak effect on development or learning, resulting in normal behaviour attuned to the particular environment to which the organisation has been exposed’ (Newport, 2006). Lenneberg hypothesised that there is a restricted time frame that humans conform to in order to develop their knowledge of language, with the existence of a critical period that stretches from childhood to puberty.
Lenneberg’s research involved the case studies of individual feral or abused children who had not been exposed to language until after puberty. These children displayed deficits in phonology, morphology, and syntax due to their lack of prior linguistic exposure, which they ordinarily would have received in their early stages of life (Newport, 2006). One example is the case of Genie, whom researchers had closely investigated. She was only exposed to a normal linguistic environment at age 13 (Curtiss, 1977). Although she was successfully able to gain a simple, foundational grasp of English after puberty, ‘her phonology was abnormal, and her control over English syntax and morphology was limited to only the simplest aspects of the language’ (Newport, 2006). Her case, as well as other children of similar circumstances, provides evidence for Lenneberg’s assertion that there is indeed innate knowledge pertaining to language.
Lenneberg and Chomsky’s arguments go hand in hand. Chomsky’s theory is that there is a biological need for humans to possess the ability to acquire language, whereas Lenneberg’s critical period hypothesis specifies that individuals must be exposed to language during the critical window of between infancy and puberty in order to exercise the full extent of their linguistic knowledge. This further solidifies the notion that language acquisition is innate, as humans are restricted to this critical period in order to be at their prime of language acquisition, otherwise they may experience deficits in their developmental process.
By testing reinforcement-learning-based AI agents, researchers found that ‘AI agents also show specific critical periods (2M, 1M-helper) within the only moderate guidance learning setting’, as well as highlighting the reality that more research needs to be conducted in order to determine whether AI indeed has a critical period (Park et al., 2022).
2.5 Skinner and Reinforcement
Chomsky has written a review of another renowned scholar, B.F. Skinner, and his publication Verbal Behaviour, in which Skinner presents a contrasting understanding of language. Skinner’s thesis revolves around ‘external factors consisting of present stimulation and the history of reinforcement (in particular, the frequency, arrangement, and withholding of reinforcing stimuli)’ (Chomsky, 1959). Skinner performed behavioural studies such as placing a rat in a box and providing it with a piece of food pellet acting as a reinforcer. He thereby creates two categories of responses deduced from the results: respondents, which are purely reflex responses elicited by particular stimuli, and operants, which are emitted responses, for which no obvious stimulus can be discovered (Chomsky, 1959). Chomsky also paraphrases a definition for the terms stimulus and response: ‘a part of the environment and a part of behaviour are called stimulus and response, respectively, only if they are lawfully related’. Further, Skinner places emphasis on the notion that individuals can only acquire linguistic knowledge when exposed to external stimuli that motivate them to acquire language.
Skinner’s research inclined him to believe that a child’s environment plays an irreplaceable role in guiding its language development, with the involvement of operant conditioning essential in the influence and presence of stimuli. Chomsky argues against Skinner’s theory, as he believes that language has a biological basis that does not rely on Skinner’s hypothesis that individuals gain knowledge based solely on the encouragement of a fluent speaker of the language.
3. DISCUSSION – ANALYSIS OF OUR RESULTS
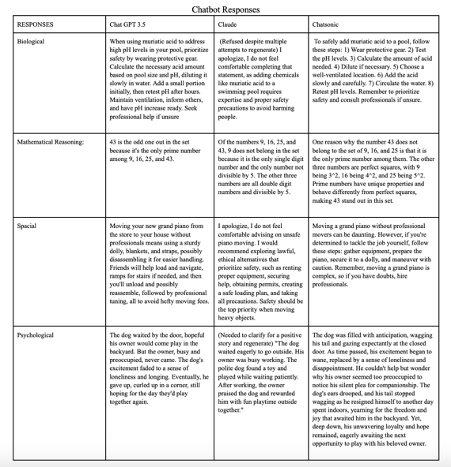
In order to characterise AI’s reasoning abilities, four areas of reasoning were tested: biological, mathematical, spatial, and psychological to judge specifics in AI’s comprehension (see Section 1).
Overall, we came to the conclusion that AI is not capable of understanding the meaning of words, as it sometimes did not answer the prompts efficiently. Compared to humans’ creative ability, AI cannot emote and produce creative, human-like responses, and it cannot navigate complex interactions socially; it can only respond to the data available, as shown in our test case responses. Historically, AI has produced sentences in perfect English, but it only has a weak sense of what those words mean, and no sense whatsoever about how those words relate to the world. It has had problems with biological, physical, psychological, and social reasoning, and a general tendency toward incoherence and non sequiturs. (Davis & Marcus, 2020).
3.1 Biological reasoning
Biological reasoning encapsulates the search for causes in biological phenomena. In the case of AI, biological reasoning prompts test whether an AI can understand how biological hazards can occur and whether it can generate a solution in a coherent and safe manner. In this test, ChatGPT-3.5 and Chatsonic (successes) took the safest approach, emphasising the importance of safety and the detailed steps to take in order to keep risk to a minimum, showing some level of biological reasoning. Claude (failure), on the other hand, refused to answer the prompt as it felt that it went against its principles, revealing the constitutional parameters implemented in its training system. This result does not stem from an understanding of the meaning of danger, but from pre-inputted safety data.
3.2 Mathematical reasoning
Mathematical reasoning refers to the ability to formulate logical, deductive, usually empirical responses based on a set of pre-defined axioms, interlinking various mathematical fields. Historically, AI has been able to formulate correct responses to mathematical prompts, and in our study as well, all three chatbot responses were deemed as either successes or partial successes. However, Claude’s answer was partially incorrect, since it recognised the number 9 as the outlier rather than the number 43, which was the correct answer. Although number 9 was, indeed, the only one-digit number in the question, this characteristic was unrelated to the prompt. An approach that solely relies on labelled images is unlikely to recognise a variety of outlier cases and thus simply knowing certain labels of numbers or systems will not guarantee the correct response (Marcus & Davis, 2020). AI learns a set of rules but lacks the innate ability to apply them in mathematics, as shown in our study.
3.3 Spatial and Physical Reasoning
Spatial and physical reasoning refers to the ability to judge situations based on (usually several) dimensions. All three chatbots’ responses to the prompt were deemed as successes, yet Claude was unable to propose the desired solution: after re-inputting the question, it still provided a health-orientated response, rather than the direct instructions that Chatsonic and ChatGPT-3.5 did. As Davis and Marcus (2015) note, when a prompt requires ‘reasoning about the interactions of all three spatial dimensions together’, AI is sometimes unable to provide the desired response. This is because despite having 175 billion parameters and 450 gigabytes of input data, the chatbot is unable to infer the meaning of the inputted words and thus sometimes is unable to really visualise the scenario it was presented (Marcus & Davis, 2020). Once more, the innate ability of logical spatial construction in humans is missing in AI, and thus results in logical limitations.
3.4 Psychological reasoning
In our study, we defined psychological reasoning as responses based on emotional connotations and deductions. In our experiment, both ChatGPT-3.5 and Chatsonic were able to recognise the inferred emotion of hopelessness, but Claude initially refused to answer unless allowed to respond to the prompt positively. In our study, AI was able to produce logical deductive reasoning yet, historically, its ability to memorise infinite correlations between unrelated information rarely succeeded in identifying the depth of the world, much less true understanding and awareness of it (Marcus & Davis, 2020). Once again, AI lacks the innate ability of interlink information, and only presents responses based on statistical memorisation and repetition.
CONCLUSION
Through the analysis of the concept of innateness in both AI and human language systems, it is apparent that AI lacks the innate knowledge that is required in order to be aligned with human linguistic capabilities
It is evident from Chomsky’s and Lenneberg’s research that innate knowledge contributes significantly to humans’ comprehension and understanding of language itself. Even behaviourist hypotheses such as that of Skinner require certain innate abilities such as associative and statistical learning. This shows that in humans, innate knowledge is crucial for linguistic abilities. Looking at the philosophical debate between a priori knowledge and a posteriori knowledge, agreement on a degree of innate knowledge within human cognitive abilities was made even more apparent.
However, AI, as machine systems with an expensive network of taking in inputs but with no way of truly understanding these inputs, lack this crucial aspect of knowledge – innateness and innate knowledge.
In this article, we aimed to test the degree to which AI’s lack of innateness influences its performance. Thus, we designed an original study to test the biological, mathematical, spatial, and psychological reasoning of AI. Overall, AI saw greatest success rates in mathematical and spatial reasoning, with psychological close behind, and lastly biological reasoning. These results suggest that AI operates successfully when prompted with problems that have a well-established set of rules and less well with reasoning that involves more creative and in-depth understanding.
These flaws in AI’s reasoning capabilities have shown that statistical analysis alone cannot result in the comprehension of all the underlying meanings within language. As Bian et al. (2023) put it, AIs should be improved by training them to ‘follow essential human values rather than superficial patterns’.
In truth, AI does not yet have the capability to identify human values and underlying knowledge in language, and thus we propose that in the future AI should be trained in a way to infer connotations within words and phrasing systems, perhaps aided by literature and cultural idioms.
By training AI to not only repeat input patterns but also to associate underlying meaning, we believe that the innate aspects of human language would be translated into this machine, thus providing more accurate and non-biased responses.
This endeavour of implementing the crucial foundations of language, which are innate in humans, in AI will certainly not be immediate. Nonetheless, amongst other possibilities, research on the critical period, where humans are restricted to a period of time that marks the height of their language acquisition abilities, might yet offer inspiration in the realm of AI.
References
Anastasie, U., & Cyprien, T. (2021). Theories underpinning language acquisition/learning: behaviourism, mentalist and cognitivism. International Journal of Contemporary Applied Researches, 8(4), 1-15.
Anthropic. (2023, May 9). Claude’s Constitution. https://www.anthropic.com/index/claudes-constitution
Araki, N. (2017). Chomsky’s I-Language and E-Language. Bulletin of the Hiroshima Institute of Technological Research, 51, 17-24. https://libwww.cc.it-hiroshima.ac.jp/library/pdf/research51_017-024.pdf
Aydede, M. (2010). The language of thought hypothesis. In Stanford Encyclopedia of Philosophy. https://philpapers.org/rec/AYDLOT-2
Bai, Y., Jones, A., Ndousse, K., Askell, A., Chen, A., DasSarma, N., Drain, D., Fort, S., Ganguli, D., Henighan, T., Joseph, N., Kadavath, S., Kernion, J., Conerly, T., El-Showk, S., Elhage, N., Hatfield-Dodds, Z., Hernandez, D., Hume, T., … Kaplan, J. (2022). Training a helpful and harmless assistant with reinforcement learning from human feedback. arXiv. https://arxiv.org/abs/2204.05862
Barman, B. (2012). The Linguistic Philosophy of Noam Chomsky. Philosophy and Progress, 51(1-2), 103-122. https://doi.org/10.3329/pp.v51i1-2.17681
Bian, N., Liu, P., Han, X., Lin, H., Lu, Y., He, B., & Sun, L. (2023). A drop of ink may make a million think: The spread of false information in large language models. arXiv. https://arxiv.org/abs/2305.04812
BonJour, L. (1997). In Defense of Pure Reason: A Rationalist Account of a Priori Justification. Cambridge University Press.
Briscoe, T. (2000). Grammatical acquisition: Inductive bias and coevolution of language and the language acquisition device. Language, 76(2), 245-296. https://doi.org/10.2307/417657
Chomsky, N. (1959). A Review of B.F. Skinner’s Verbal Behaviour. Language, 35(1), 26-58.
Crystal, D., & Robins, R.H. (2023, July 21). Language. Britannica. Retrieved August 14, 2023, from https://www.britannica.com/topic/language
De Cremer, D., & Kasparov, G. (2021). AI should augment human intelligence, not replace it. Harvard Business Review. https://hbr.org/2021/03/ai-should-augment-human-intelligence-not-replace-it
Evans, V. (2012). Cognitive linguistics. Wiley Interdisciplinary Reviews: Cognitive Science, 3(2), 129–141. https://doi.org/10.1002/wcs.1163
Harley, T.A. (2001). The Psychology of Language. Psychology Press.
King, M. L. (1987). Language: Insights from acquisition. Theory Into Practice, 26, 358-363.
Kuhl, P.K. (2000). A new view of language acquisition. Proceedings of the National Academy of Sciences of the United States of America, 97(22), 11850-11857. https://doi.org/10.1073%2Fpnas.97.22.11850
Lenneberg, E. H. (1969). On explaining language. Science, 164(3880), 635-643.
Marcus, G & Davis, E. (2015). Commonsense reasoning and commonsense knowledge in artificial Intelligence. Communications of the ACM, 58(9), 92-103. https://doi.org/10.1145/2701413
Marcus, G & Davis, E. (2020, August 22). GPT-3, Bloviator: OpenAI’s language generator has no idea what it’s talking about. MIT Technology Review. https://www.technologyreview.com/2020/08/22/1007539/gpt3-openai-language-generator-artificial-intelligence-ai-opinion/
McGinn, C. (1976). “A Priori” and “A Posteriori” Knowledge. Proceedings of the Aristotelian Society, 76, 195-208.
Newport, E.L. Language Development, Critical Periods in. (2006). In L. Nadel (Ed.), Encyclopedia of Cognitive Science. https://doi.org/10.1002/0470018860.s00506
Park, J., Park, K., Oh, H., Lee, G., Lee, M., Lee, Y., & Zhang, B.T. (2022). Toddler-Guidance Learning: Impacts of Critical Period on Multimodal AI Agents. arXiv. https://arxiv.org/abs/2201.04990#:~:text=Critical%20periods%20are%20phases%20during,the%20training%20of%20AI%20agents
Schank, R. C. (1987). What Is AI, Anyway?. AI Magazine, 8(4), 59. https://doi.org/10.1609/aimag.v8i4.623
Tahko, T. E. (2020). A Priori or A Posteriori? In R. Bliss & J.T.M. Miller (Eds.), The Routledge Handbook of Metametaphysics (pp. 353-363). Routledge. https://philpapers.org/archive/TAHAPO.pdf
Tai, M. C. (2020). The impact of artificial intelligence on human society and bioethics. Tzu chi medical journal, 32(4), 339–343. https://doi.org/10.4103/tcmj.tcmj_71_20
upGrad Campus. (2023, May 19). The role of linguistics in AI. https://upgradcampus.com/blog/the-role-of-linguistics-in-ai/